Technicity of listening
The first workshop in Radio explorations addressed the technicity of listening: knowledge, tools and approaches to sonic and other data. Three invited guests, Carl Colena (SIGID wiki), Miro Roman (CAAD, ETHZ) and Simone Conforti (IRCAM) presented their current research into archives of radio signals, images, books and music, as well as strategies for articulating profiles and identities with data. Technicity of listening to radio signals, listening to data streams of images and listening to music, as well as the depths of these concerns correspond with the depth of issues that we are facing in this project. The three different listening aspects that we discussed demonstrate three possible planes of concern, which determine the volumetric scope of this research. In the space that is marked by the three interests, the Radio explorations team presented the first prototype of the Codebook: the exploration of the archive of radio signal recordings, translated to sound and organized with self-organizing map (SOM) machine learning algorithm.
Carl Colena: Listening to Radio Signals
Carl Colena talked about his interest in signal identification, which started with his studies in computer engineering at the City College of New York and the discovery of the RTL-SDR affordable computer-based radio scanners. Over the years, it became both his hobby and profession. The community of users around the SIGID wiki use the website for a variety of purposes. There are amateur radio operators who focus on shortwave transmissions (SWLs), listening to radio to discover new signals and track the patterns of different transmissions in their area. Other users come from the information security space, seeking to understand more on wireless and baseline some of their designs. Then there are tech hobbyists who are interested in the radio space in general, in radio frequency and the ability to transmit information in various different ways. What are radio signals and how are they received? How do signals coexist in time and space? How can we judge the intentionality of certain transmissions?
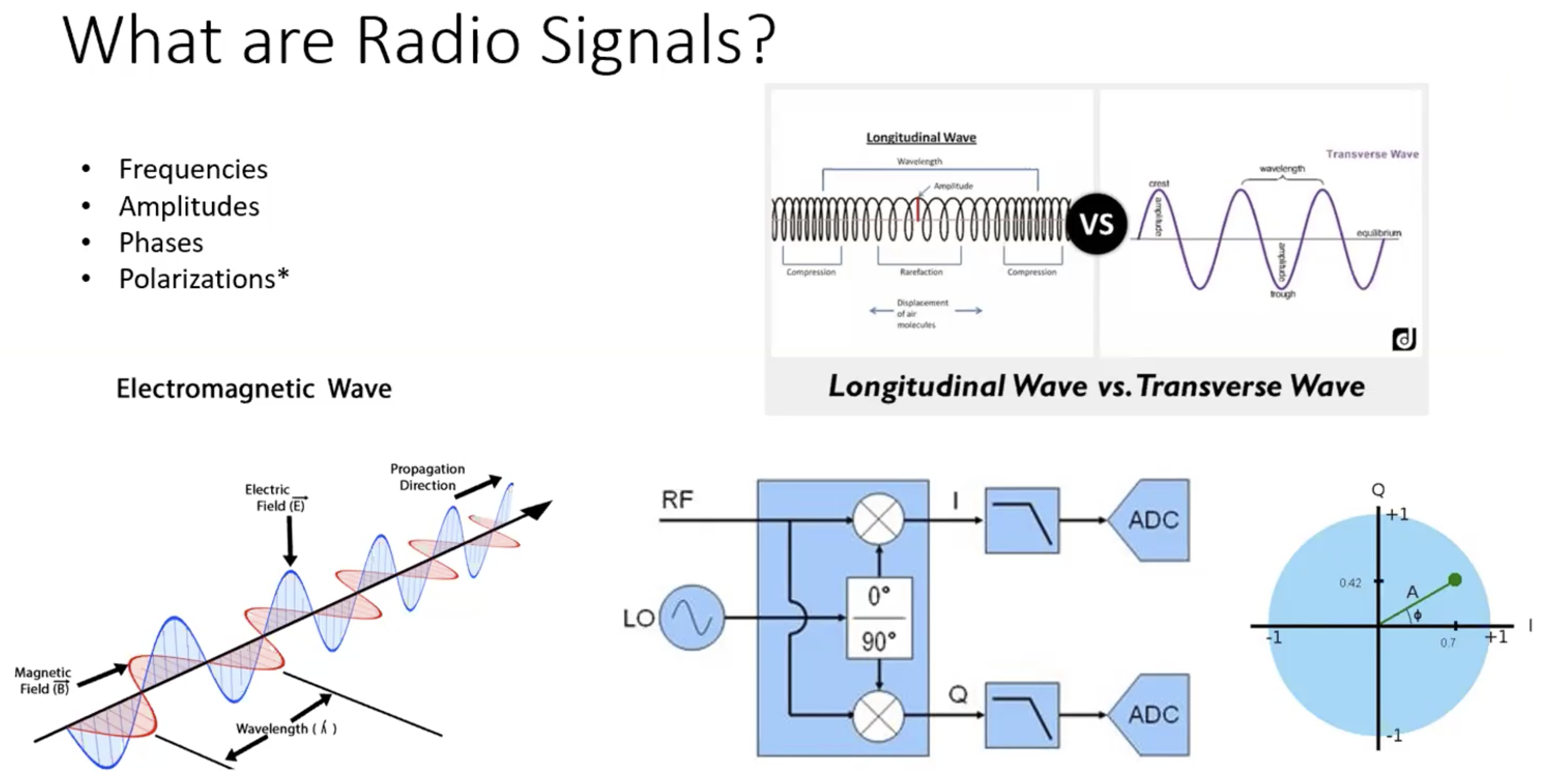
Physical properties of radio and acoustic waves. Courtesy of Carl Colena
Colena shared his extensive knowledge on these and other questions related to communication engineering in the context of radio signals. The knowledge practices of the community of radio enthusiasts gathered around the SIGID wiki website are based on acoustic and visual interpretations of signals using different software tools. It is about looking for patterns, signatures and tonalities, combined with the visual component. It is important to have multiple perspectives on the signal at once. A variety of modes, for example the upper-side band, AM and FM are often, depending on the way that the signal was intended to be received.
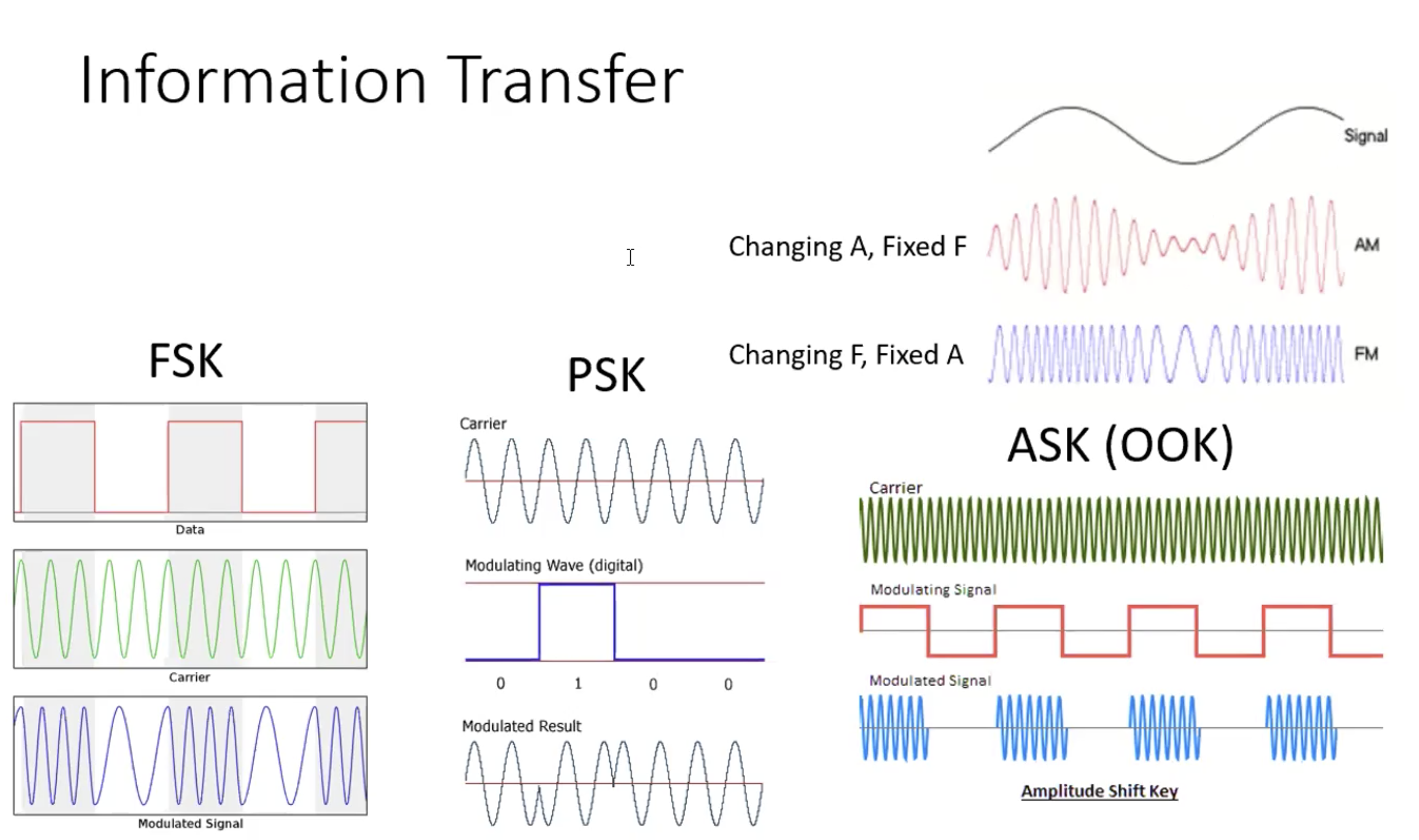
Three common digital methods for data transmission: frequency shift keying (FSK), phase shift keying (PSK) and amplitude shift keying or on-off keying (ASK/OOK). Courtesy of Carl Colena
Natural radio is also the object of listening practices. Several natural phenomena produce radio signals. Media scattering is a technique of listening for reflections of man-made transmissions off of the ionizing particle cloud of a passing meteorite. More frequent natural phenomena include spherics and whistlers, purely natural transmissions induced by natural events, such as lightning and its reverberation.
Finally, to find a common perspective to observe all signals in the archive, Colena proposed several forms of translating and compressing the data. Autocorrelation function (ACF) can compress the frequency of a modulating signal into a single number, if a signal has that sort of periodicity. Another attribute could be the minimum spacing between the tones of frequency shift keying (FSK). In signals that are not well structured, such as natural phenomena, it may hard to find a regularity. To address signals more generally requires looking for signal differentiation: taking a derivative of the signal or looking at the rate of change of different properties throughout. This means to find a way to ‘signaturize’ signals, come up with some sort of categorisation that can be used on all signals, a way to encode the dataset.
Miro Roman: Spelling Alice. Pixels, Voxels and Atom-letters
Miro Roman, architect and researcher working at the Digital Architectonics Chair of Professor Hovestadt in Zürich, ETHZ presented an articulation of his interest in architecture and information technology. How can we think about architecture through notions of information, big data, and computers? Roman introduced his story of Alice, an avatar character that at once encodes and expresses his way to work with abundant digital information, such as images on architecture and design blogs and social media. Roman approaches the question of coding and computation from the concept of literacy: coding on a level which is not about problem solving but about articulating and seeing character in data.
Alice starts in a noisy space, with all the images of ArchDaily and Dezeen in the past ten years. It is counted in millions, and it is only getting more. These are then brought to a space of numbers through different encoding processes: transforming images into same resolution and reading out RGB values as lists; the frequency of structure in image parts (edge detection); measuring overall entropy of the image; extracting specific features. use the self-organizing map (SOM) machine learning algorithm for clustering. I take all the images of ArchDaily and Dezeen, and make a cloud, a weather of architecture in the past ten years, an impression or a face of it. It speaks of what has been happening in architecture in terms of weather. I can get a structure of images based on the colour of pixels, clustering images by structure and by form without having any input on what these elements mean. If we then take any two images, for instance a chair and something else, there will be infinite ways how we can relate them. We can relate them through the shortest path between them; or we can find random curves to relate them. The way we choose to relate the two images will be our story. The SOM algorithm clusters these images in a sort of a cloud, a weather or a face of design and architecture in social media. Out of the weather, Roman takes specific flavours and brings them into a profile, which can be about textures, or drawings, a particular colour, shape or object.
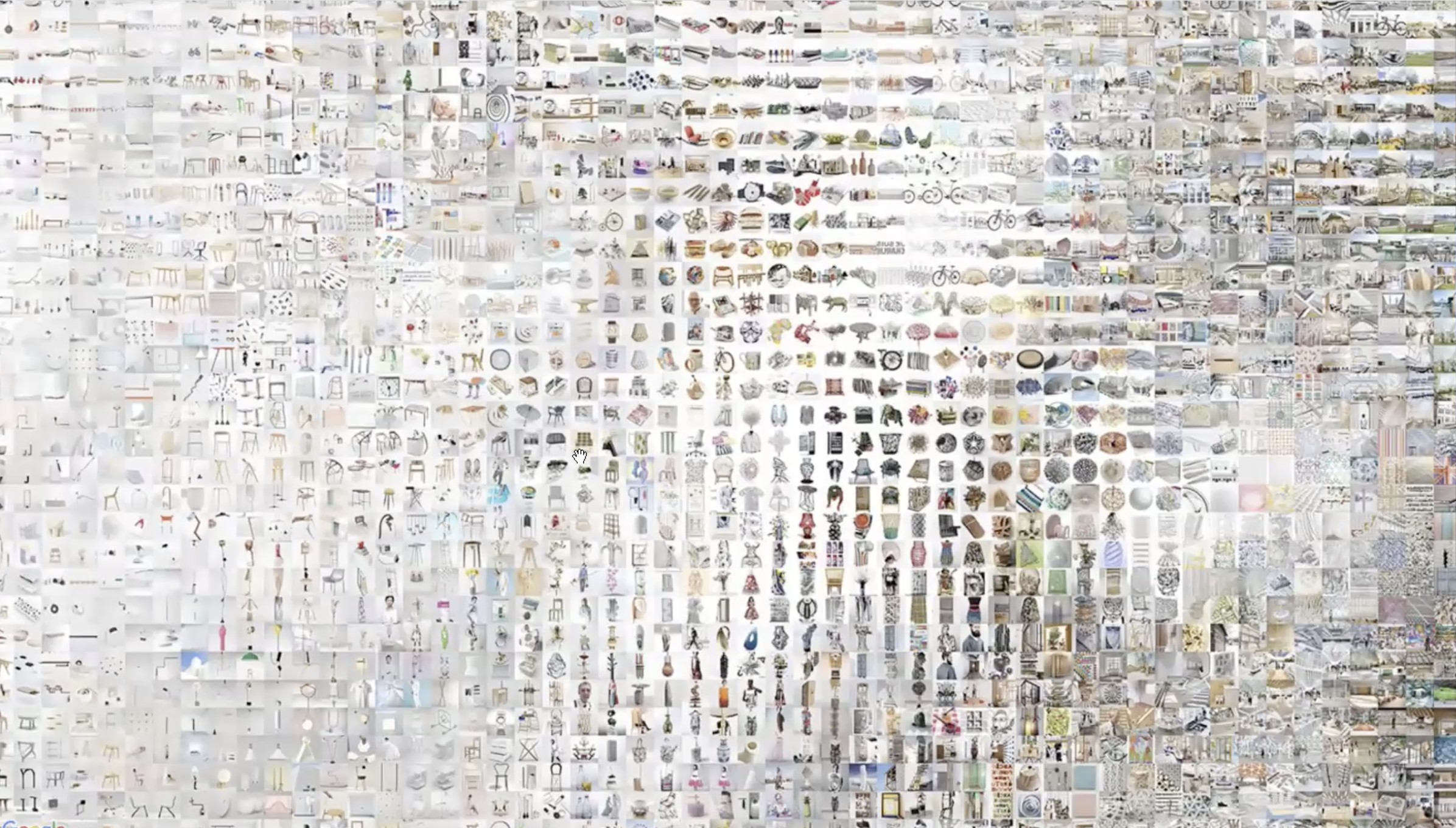
Weather of images from Dezeen. Courtesy of Miro Roman
Another field of interest Roman explored with Alice is writing on architecture and how it is reflected in books in general. With a ‘brain’ made of words - a galaxy of concepts, and a body made of books clustered according to the way words describe specific collections of books (word counting in terms of natural language processing and information retrieval), Alice can now tell about a specific concept, such as ‘information’, in this specific setting - not a generic definition of information, but as it is situated in this specific archive of text. It is about understanding information in the context of electricity and atoms: a kind of electric information. This speaks of articulating things in their own terms: when we are in a specific library, then we take only the words that really appear in its texts.
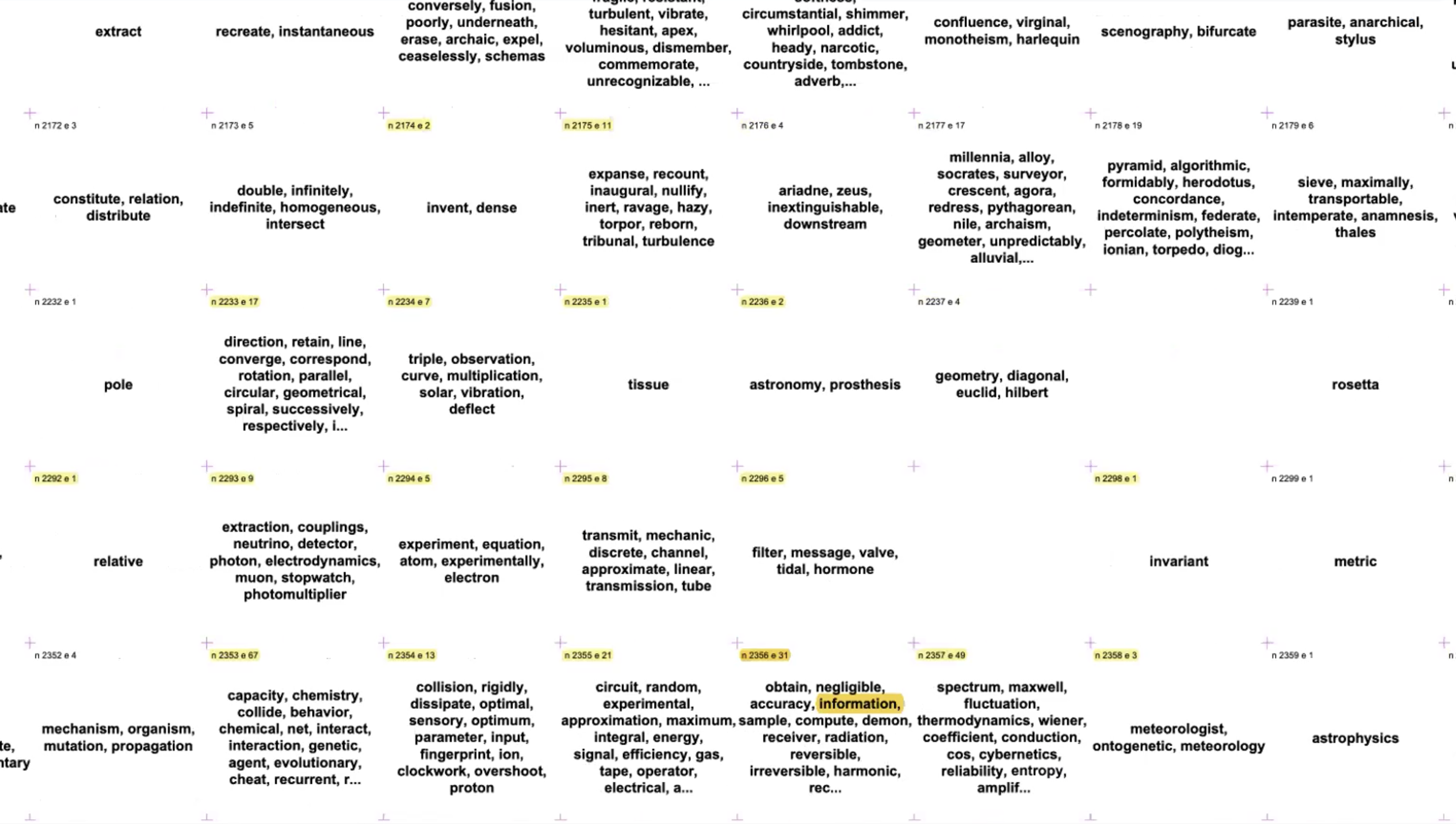
Galaxy of concepts and articulation of the term ‘information’. Courtesy of Miro Roman
Based on these techniques, Alice can also host a computer-mediated conversation. We can choose a topic, for example ‘information’, a specific library to search, and a statement, for example ‘articulating an object in own terms’. Alice returns sentences from books which a related to the statement in a meaningful way.
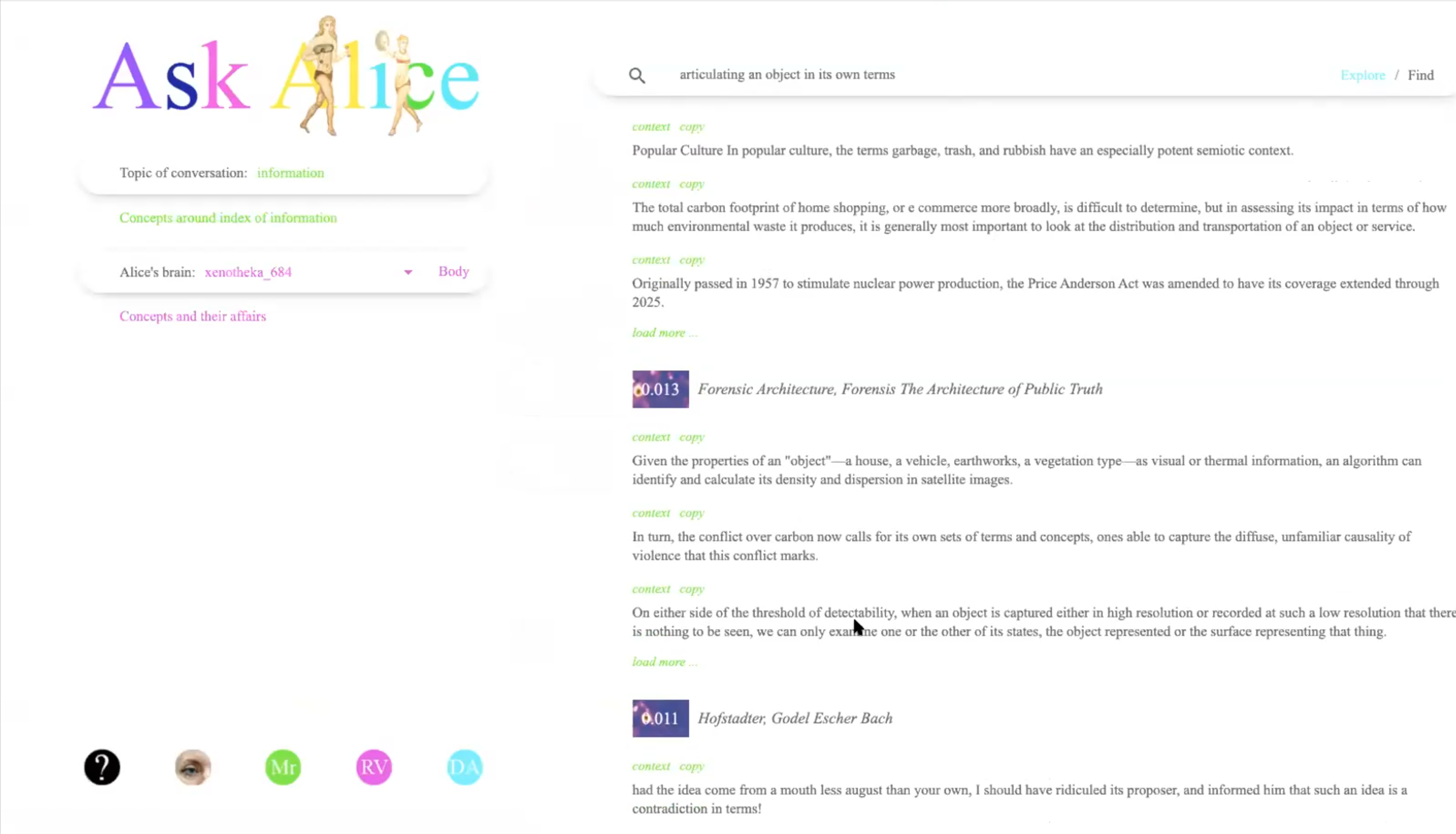
Asking Alice about information and articulating an object in own terms. Courtesy of Miro Roman
Articulations of images and texts in terms of specific archives give rise to a synthetic character that is able to comment on images using a platform such as Twitter. The character of Alice takes random images from the archive, interprets their content and relates it to the libraries. She posts these combinations regularly, under the handle @Alice_ch_n3e81. Alice is a character, able to act and talk, but unlike regular actors, she operates without a given script. She can find different ways of relating information. She is a character via which one can navigate the internet and play with these informational techniques.
Simone Conforti: Actual Listening and Automatic Sonic Segmentation
Simone Conforti presented his research into classification of sonic sources, conducted in the context of the SNF-Sinergia research project Radiophonic Cultures (2015-2019). This project is an attempt to articulate the idea of actual listening with neural networks of machine learning algorithms. The actual listening approach is close to how humans listen to sonic facts, which preserves a memory of the longer time span and therefore a context for any individual event. Conforti worked with the German experimental radio archive held at the Weimar University, exploring way to classify different sonic sources such as a radio talk show, dance music, a singing female voice, and the piano.
In this research, attuned at finding ways to characterize sound, sonic information is compressed using the bark scale representation1, which corresponds to the subdivision of hair cells in our inner ear (cochlea). Neural network trained on this data searches for changes in a continuous mix of different sonic content: from talking with background music, to dance music, then a solo female singing voice, to solo piano playing Satie. The tool prototype creates a step map of cosine similarities across the duration of the mix, and compares these at bark scale. It is able to identify points in which sound content changes significantly.
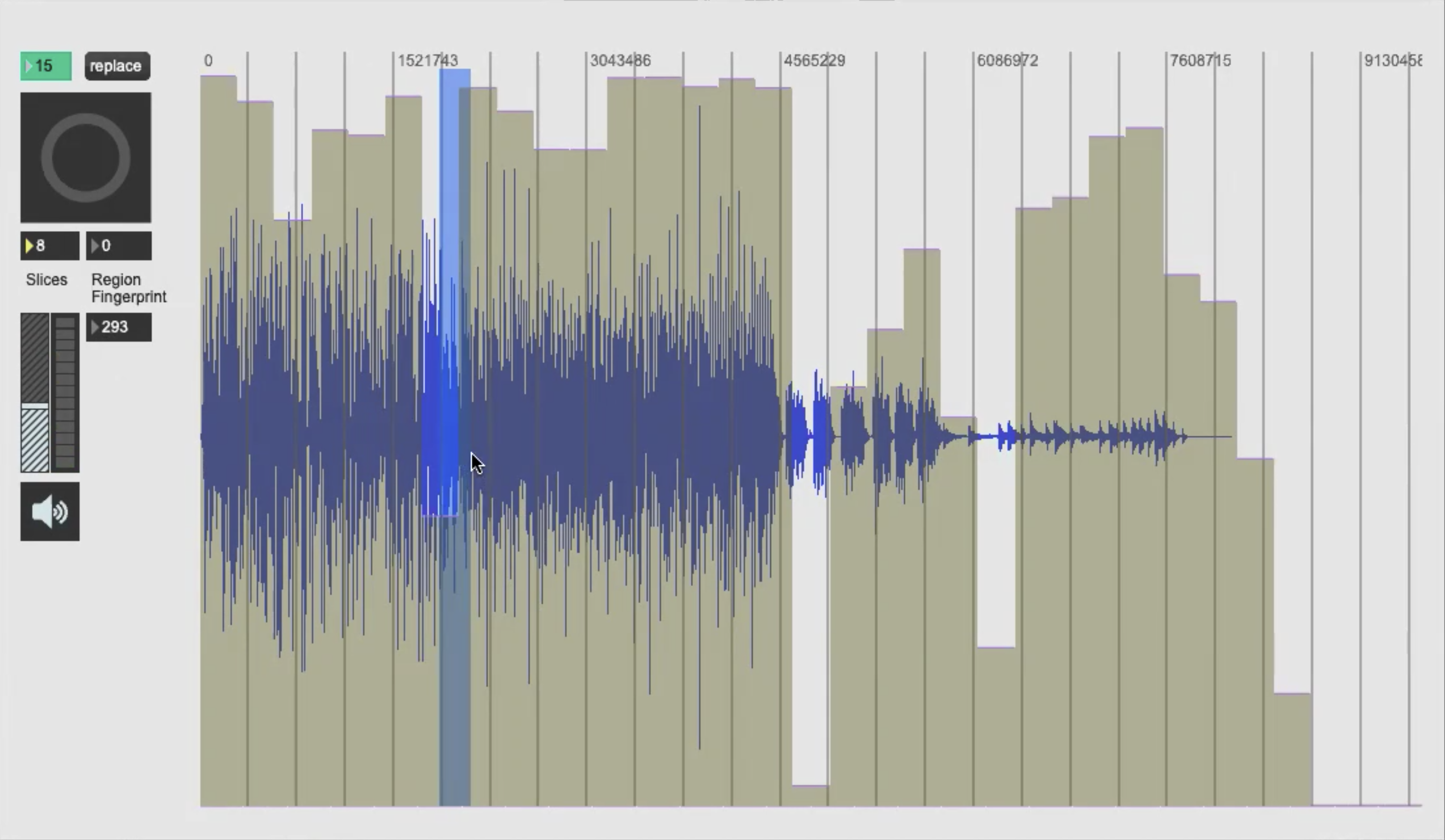
The prototype tool for identification of changes in the mix (low bars). Courtesy of Simone Conforti
The idea is that we can use these extracted vectors, these fingerprints as the training set for a self-organizing map. I would use these region fingerprints as the way to organize my map and afterwards I can use whatever input vector as a bark scale vector to classify a new sound object.
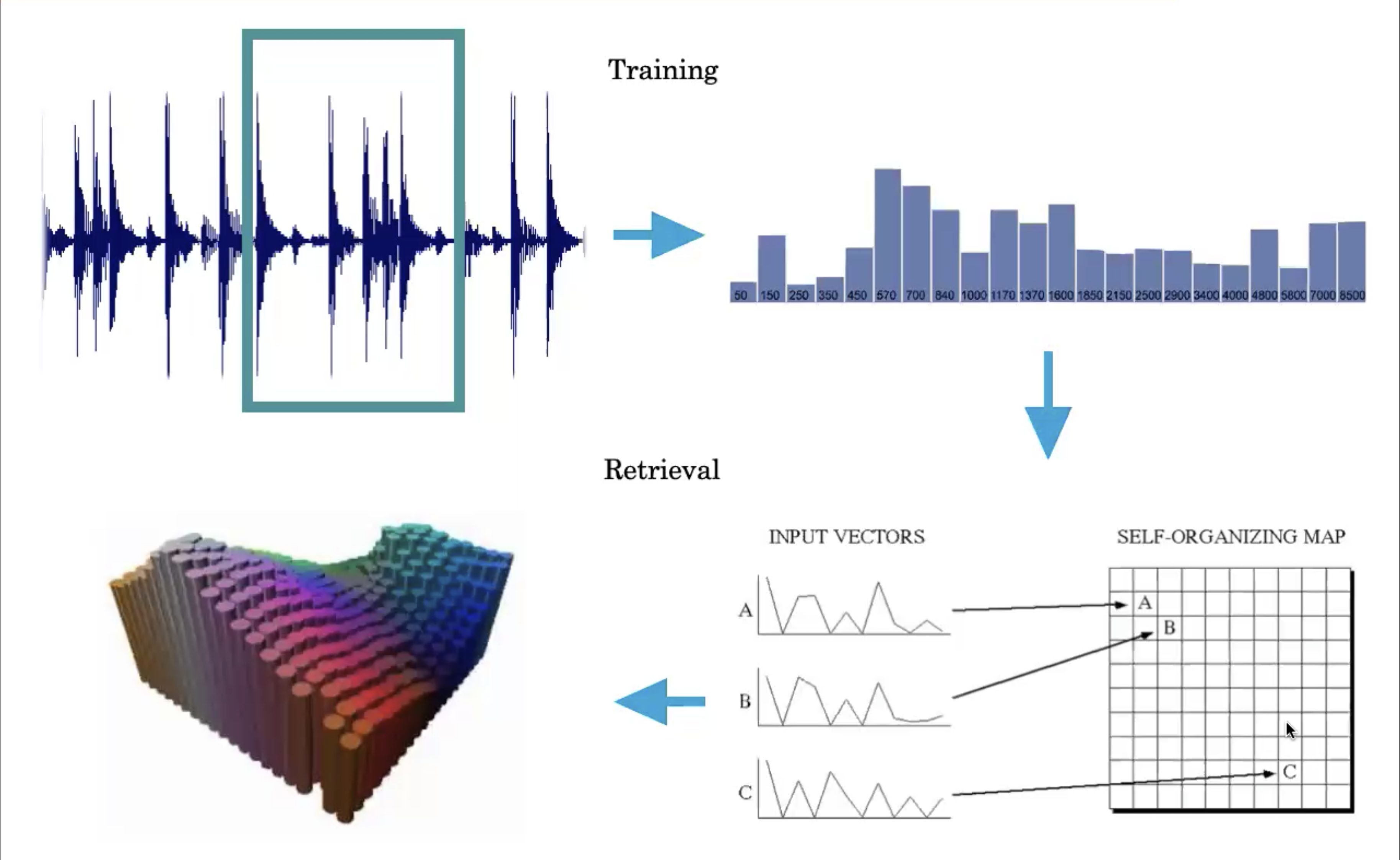
From sound to SOM. Courtesy of Simone Conforti
Conforti also discussed possible low-level descriptors for radio signals, beyond spectrograms and fingerprints used so far. Noise in the radio signal can come from interferences in the transmission, but also be part of the transmission. Different sounds have patterns, which have different lengths. Spectral centroid and spread can be useful because those are characterizing not the frequency in itself, but the energy of the sound: the very centre of the spectrum and the distribution. Furthermore, onset detection, and analysis of evolution of a sound within a timeframe could be used. All these could be taken into account when trying to define or identify a sound, or a radio signal.
-
Eberhard Zwicker, Subdivision of the Audible Frequency Range into Critical Bands, Journal of the Acoustical Society of America 33, no. 2 (1961): 248, https://doi.org/10.1121/1.1908630. ↩︎